Researchers from Michigan Tech and the University of California, Los Angeles (UCLA) are collaborating on a machine learning model that aims to break the cubic scaling barrier of quantum mechanics.
Kohn-Sham density functional theory (KSDFT) is the prevalent method of determining electronic structure in materials science, physics, and chemistry. It's prized for accuracy. But there are cost-scaling challenges for researchers seeking predictive simulations of massive and intricate molecular systems. KSDFT calculations for these systems take too long and are too computationally expensive to be feasible.
Using artificial intelligence and machine learning algorithms, engineers and scientists have developed a new model to accurately and much more efficiently predict the electronic structure. Susanta Ghosh, an assistant professor in the Department of Mechanical and Aerospace Engineering, said the new model has the potential to expand—and transform—the scientific capacity to accelerate materials research, opening avenues for future groundbreaking discoveries.
"Not only is our new model exceptionally accurate, it significantly accelerates computations compared to traditional KSDFT," said Ghosh. "The result is that simulations of even multimillion-atom systems are now attainable."
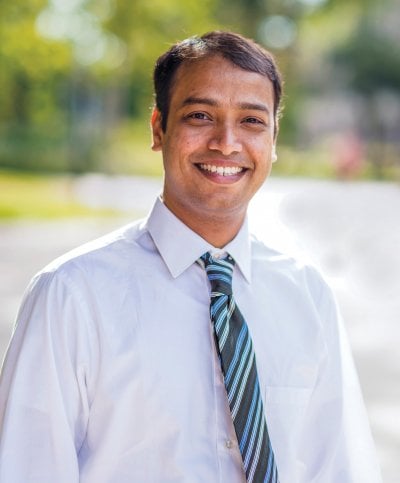
Ghosh and then-PhD students Shashank Pathrudkar and Ponkrshnan Thiagarajan collaborated with Shivang Agarwal and Amartya S. Banerjee of UCLA to develop the new machine learning model through research conducted by Ghosh's group at Michigan Tech and Banerjee's at UCLA.
The results, titled "Electronic Structure Prediction of Multi-million Atom Systems Through Uncertainty Quantification Enabled Transfer Learning," have been published in npj Computational Materials.
"Machine learning has helped push the envelope of large-scale first-principles calculations significantly," said Banerjee, lead author on the paper and assistant professor in the Department of Materials Science and Engineering at UCLA.
"When I was a postdoctoral fellow some years ago, we used cutting-edge computational techniques on some of the largest supercomputers in the country to calculate the electronic structure of bulk systems containing tens of thousands of atoms," Banerjee said. "A typical calculation would take many hours or even days, on tens of thousands of processors. Now, thanks to our work, we can do similar calculations for millions of atoms on a single GPU, in a matter of a few hours. Fundamentally, these machine learning techniques appear to solve the equations of quantum mechanics in a way that is quite different from what we conventionally do as humans. The interesting scientific problem is figuring out what that is."
In the realm of physics, chemistry, and materials science, first-principles calculations that quantify physical properties directly from basic physical qualities are the cornerstone of computational methods. Techniques delve deep into the fundamental laws that govern a system's properties and behaviors, drawing from the bedrock of quantum mechanics. The calculations solve equations that encapsulate a material's behavior, similar to the intricate Schrödinger equation that governs electrons within atoms or molecules.
In computational materials science, KSDFT has long been the go-to method for predicting material properties and discovering new materials. But its ability to simulate material systems with a large number of atoms is limited. For certain applications, such as complex materials or multimillion-atom systems, the use of routine KSDFT isn't feasible. Researchers find themselves grappling with expensive, challenging, and sometimes impossible experiments.
The technique becomes more expensive, demanding more computational time and memory requirements, as the number of atoms in a material sample increases. These limitations restrict routine KSDFT calculations to just a few hundred atoms.
The electronic structure of a material is a fundamental quantity that contains a wealth of information, said Ghosh. "It can be used to predict an array of important material properties. Accordingly, this new model is not restricted to any particular property of interest; it can predict the electronic structure first—and then subsequently derive any material property," he said.
Ghosh, who heads the Computational Science and Machine Learning Lab at Michigan Tech, served as faculty advisor for both Thiagarajan and Pathrudkar
Thiagarajan is currently a postdoctoral fellow at John Hopkins University, and Pathrudkar is now a senior engineer at MathWorks.
Pathrudkar said he focused his efforts on research at the intersection of machine learning, mechanics, and material science during his six years working with Ghosh. "The overarching goal of my research while at Tech has been to leverage machine learning models to bypass computationally expensive materials research methods," said Pathrudkar. "The application of artificial intelligence in materials science has demonstrated remarkable potential in discovering novel materials that could revolutionize critical sectors such as energy harnessing, aerospace, electronics, and medical devices."
Michigan Technological University is a public research university founded in 1885 in Houghton, Michigan, and is home to nearly 7,500 students from more than 60 countries around the world. Consistently ranked among the best universities in the country for return on investment, Michigan’s flagship technological university offers more than 120 undergraduate and graduate degree programs in science and technology, engineering, computing, forestry, business, health professions, humanities, mathematics, social sciences, and the arts. The rural campus is situated just miles from Lake Superior in Michigan's Upper Peninsula, offering year-round opportunities for outdoor adventure.