Problem
Existing data sets for evaluating algorithms for detecting, classifying, and tracking pedestrians and cyclists only contain optical imagery. Even in these evaluation data sets, there is no independent measurement of their locations and trajectories. Refs [2-5]
Project Goal
Develop a set of empirical data (camera, radar, and lidar) suitable for evaluation of systems to detect, classify, and track pedestrians and cyclists.
Approach
Collect multi-sensor data with an autonomous vehicle, and independently collect measurements of other actors in the scene, concurrently with real-time kinetic corrected satellite navigation systems. Refs [6-10]
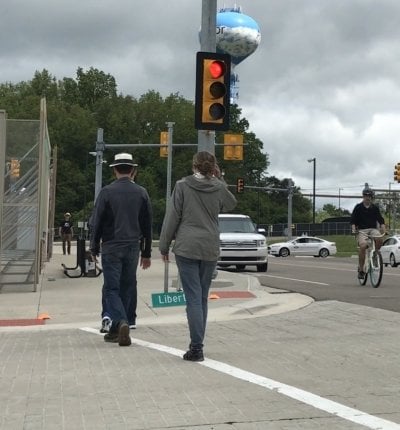
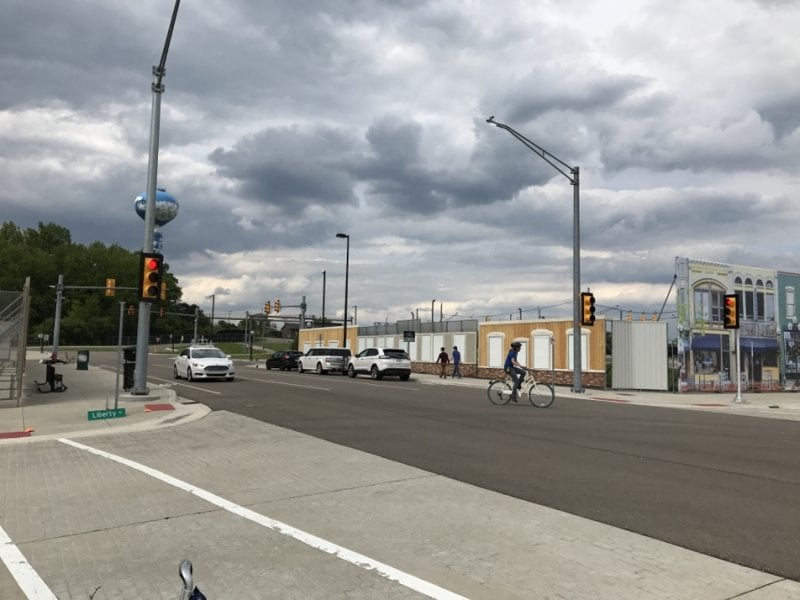
Impact
The data set can be used by researchers to evaluate the performance of algorithms against the data set and compare detections, classifications and tracks, against ground truth solutions.
SAE International World Congress April 10-12, 2018, Detroit, Michigan, USA
Abstract
The objective of this research was to collect measurements from a suite of sensors, selected for an autonomous vehicle, along with ground truth data, which can be used for the development, and evaluation of algorithms. The ground truth data was collected independently of the vehicle sensors to allow for an objective evaluation of system performance. In a variety of scenarios, designed to incorporate real world interactions of vehicles with bicyclists and pedestrians, measurements were collected by the sensor suites onboard multiple autonomous vehicles. Location measurements of the bicyclists and pedestrians were collected by separate means. All the measurements are synchronized to Coordinated Universal Time (UTC). In most cases, the real-time kinetic receivers on the bicyclists and pedestrians achieve RTK-fixed, or RTK-float accuracy, resulting in errors on the order of a few centimeters, or a few decimeters, respectively.
References
Zhang, Shanshan, Rodrigo Benenson, Mohamed Omran, Jan Hosang, and Bernt Schiele. 2017. “Towards Reaching Human Performance in Pedestrian Detection.” IEEE Transactions on Pattern Analysis and Machine Intelligence. doi:10.1109/TPAMI.2017.2700460.
Benenson, Rodrigo, Mohamed Omran, Jan Hosang, and Bernt Schiele. 2015. “Ten Years of Pedestrian Detection, What Have We Learned?” In Lecture Notes in Computer Science (Including Subseries Lecture Notes in Artificial Intelligence and Lecture Notes in Bioinformatics). doi:10.1007/978-3-319-16181-5_47.
Chakraborty, Avishek, Victor Stamatescu, Sebastien C Wong, Grant Wigley, and David Kearney. n.d. “A Data Set for Evaluating the Performance of Multi-Class Multi-Object Video Tracking.”
Dollár, Piotr, Christian Wojek, Bernt Schiele, and Pietro Perona. n.d. “Pedestrian Detection: A Benchmark.”
Zhang, Shanshan, Rodrigo Benenson, Mohamed Omran, Jan Hosang, and Bernt Schiele. 2016. “How Far Are We from Solving Pedestrian Detection?” doi:10.1109/CVPR.2016.141.
Li, T, and J Wang. 2012. “Some Remarks on GNSS Integer Ambiguity Validation Methods.” Survey Review. doi:10.1179/1752270611Y.0000000027.
Piotraschke, Hagen F. n.d. “RTK Für Arme -Hochpräzise GNSS-Anwendungen Mit Den Kostengünstigsten Trägerphasen-Rohdatenempfängern.”
Psychas, Dimitrios-Vasileios. n.d. “Accuracy Improvement Techniques in Precise Point Positioning Method Using Multiple GNSS Constellations.” doi:10.13140/RG.2.1.1386.5365.
Wisniewski, Bartosz, Krzysztof Bruniecki, and Marek Moszynski. 2013. “Evaluation of RTKLIB’s Positioning Accuracy Using Low-Cost GNSS Receiver and ASG-EUPOS.” TransNav, the International Journal on Marine Navigation and Safety of Sea Transportation. doi:10.12716/1001.07.01.10.
“PPP Ambiguity Resolution Implementation in RTKLIB v 2.4.2 Implementation in RTKLIB v 2.4.2.” n.d.