Improving the Great Lakes Operational Forecast System by creating a data assimilation and model adjustment loop.
Though the Great Lakes are called lakes, because of their sheer size they are truly inland seas. They affect regional weather patterns, provide drinking water to millions of people and drive the economies of several states.
Forecasting the water levels, temperatures and currents of the lakes is highly important because of the myriad ways lake conditions affect commerce, recreation and community well-being. These forecasts comprise the Great Lakes Operational Forecast System (GLOFS), an automated model-based prediction system operated by the National Oceanic and Atmospheric Administration (NOAA).
“The system information allows decision makers to make informed decisions and the forecast products have been used by a wide variety of users on a regular basis,” said Philip Chu, supervisory physical scientist of the integrated physical and ecological modeling and forecasting branch of NOAA’s Great Lakes Environmental Research Laboratory (GLERL).
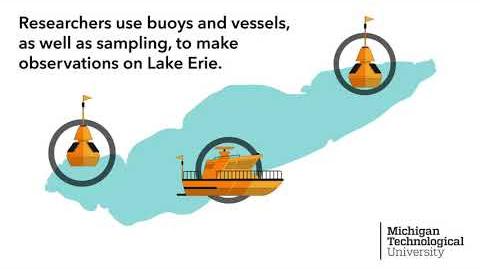
Building a Better Great Lakes Forecasting System
“Water levels are used by power authorities; wave and currents conditions are used by the U.S. Coast Guard for search and rescue missions and temperature profiles have been used by recreational boaters and fishermen,” he said. “The information has also been used to predict harmful algal blooms as well as hypoxia (low dissolved oxygen) conditions in the Great Lakes.”
About the Researcher
While NOAA operates its own modeling team to maintain the system, the agency also works with university researchers to continually improve GLOFS. At Michigan Technological University, Pengfei Xue, associate professor of civil and environmental engineering and director of the Numerical Geophysical Fluid Dynamics Laboratory at the Great Lakes Research Center, is aiding NOAA by adding a data assimilation component.
Xue noted that a typical operational forecast system should include three components: modeling, an observation network and data analysis.
“The Great Lakes region has relatively dense and long-term observational data, but how do we use the data to improve forecasting?” Xue posed. “These data have been used for model initialization and verification, but there can be a much stronger linkage between in-the-field observations and numerical modeling. Blending observational data into the model can improve short-term forecasting. This technique, called data assimilation, is one of the most effective approaches for statistically combining observational data and model dynamics to provide the best estimate of the Great Lakes system state.”
What is Data Assimilation?
To explain data assimilation, Xue gave the example of taking the temperature of a lake. A computer model might predict the temperature at a site in the lake is 68 degrees Fahrenheit (20 degrees Celsius). But a physical measurement at the site shows the temperature is 70 degrees Fahrenheit (21.1 degrees Celsius).
“All models contain some uncertainties and the observation also has noise, which can be large or small in fieldwork, depending on different cases,” Xue said. “Which should you believe? Your best bet is something in between. When we quantify the model and the observation uncertainties by assessing their historical performances, we can quantitatively combine the observational data and the numerical model results with different weights and give a more accurate estimate.”
Computer modeling is much more complicated than this example, Xue noted. One key advantage of a model, especially in a large and complex environment like the Great Lakes, is that it can produce continuous fields in 3D space, predicting — at any time and any place — temperature, water levels, and currents. On the other hand, in situ observations provide “ground truth,” but they are often limited in time and space.
“Quantifying the model and observation uncertainties is at the heart of data assimilation techniques,” Xue explained. “The beauty of data assimilation is to use the information of the misfits between the model results and observations, which are only known at limited observation locations, to correct model bias in a 3D space beyond the observation locations. Hence, it improves model accuracy for the entire simulation fields.”
More than a Model
Another limit of in-the-field observations is the sheer cost of doing them. Observational data is inherently more accurate than a model alone, and ground truthing the output of a model is necessary. By feeding observational data into a model, then using the model to predict better locations for future in situ data collection, Xue’s work helps the GLOFS modeling improve, and helps scientists choose research sites effectively.
Grants and Funding
Funding for this project was provided by the Great Lakes Restoration Initiative, through the University of Michigan Cooperative Institute for Great Lakes Research (CIGLR) cooperative agreement with the National Oceanic and Atmospheric Administration (NA17OAR4320152).
“The Great Lakes have vast surface area and great depth. Typically, where people choose to sample is based on expert empirical experience and their research interests,” Xue said. “In situ observations, particularly subsurface measurements, remain limited due to the high costs of building and maintaining observing networks. Using data assimilation to guide the design of data sampling location and frequency and optimize an observational network is one of the key research topics of an integrated observing and forecasting system.”
Xue’s preliminary results show data assimilation is able to reduce sampling efforts and increases forecasting accuracy by optimizing sampling locations.
“Professor Xue’s contribution aligns perfectly with NOAA and GLERL’s short-term goal and long-term mission on building an integrated environmental modeling system and a weather-ready nation, healthy oceans and coasts,” Chu said. “His research contribution and collaboration with NOAA scientists advance our overall understanding of the complicated dynamic system in the Great Lakes as well as accelerate NOAA’s pace to develop, improve and transition the next-generation Great Lakes Operational Forecasting System to operations.”
Xue’s work utilizes the Superior, a high-performance computing infrastructure at Michigan Tech, to build high-fidelity models. Model results are being used to build a long-term, data assimilative temperature database for Lake Erie for use by resource managers and researchers in the Great Lakes community. The Lake Erie simulation is a proof of concept prior to GLOFS being entirely refitted using data assimilation. Xue’s project will also apply machine learning to further enhance model performance and adaptive in situ sampling, with the goal to extend the method to all five Great Lakes.
“We want to demonstrate the potential of this approach. Lake Erie has experienced substantial environmental issues for decades and has been studied more comprehensively, and people realize better the modeling deficiencies,” Xue said. “The thermal structure and circulation of Lake Erie greatly impact harmful algal blooms and hypoxia events. Our plan is to gradually expand and build a fully operational forecast system with data assimilation capabilities to improve short-term forecasting accuracy and refine the observing work.”
Xue has received new funding to expand the project, entitled, “The Cooperative Institute for Great Lakes Research (CIGLR): Long-Term Data Assimilative, Temperature and Currents Database for the Great Lakes (Year 2: Lake Michigan).”
Michigan Technological University is an R1 public research university founded in 1885 in Houghton, and is home to nearly 7,500 students from more than 60 countries around the world. Consistently ranked among the best universities in the country for return on investment, Michigan's flagship technological university offers more than 120 undergraduate and graduate degree programs in science and technology, engineering, computing, forestry, business, health professions, humanities, mathematics, social sciences, and the arts. The rural campus is situated just miles from Lake Superior in Michigan's Upper Peninsula, offering year-round opportunities for outdoor adventure.
Comments